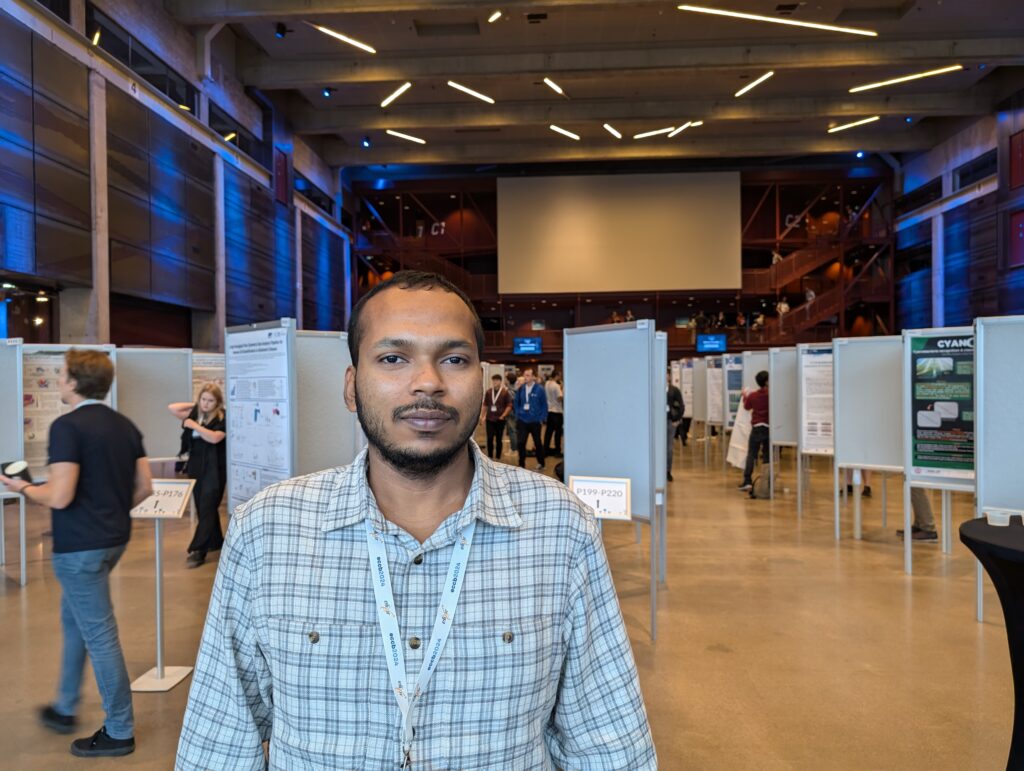
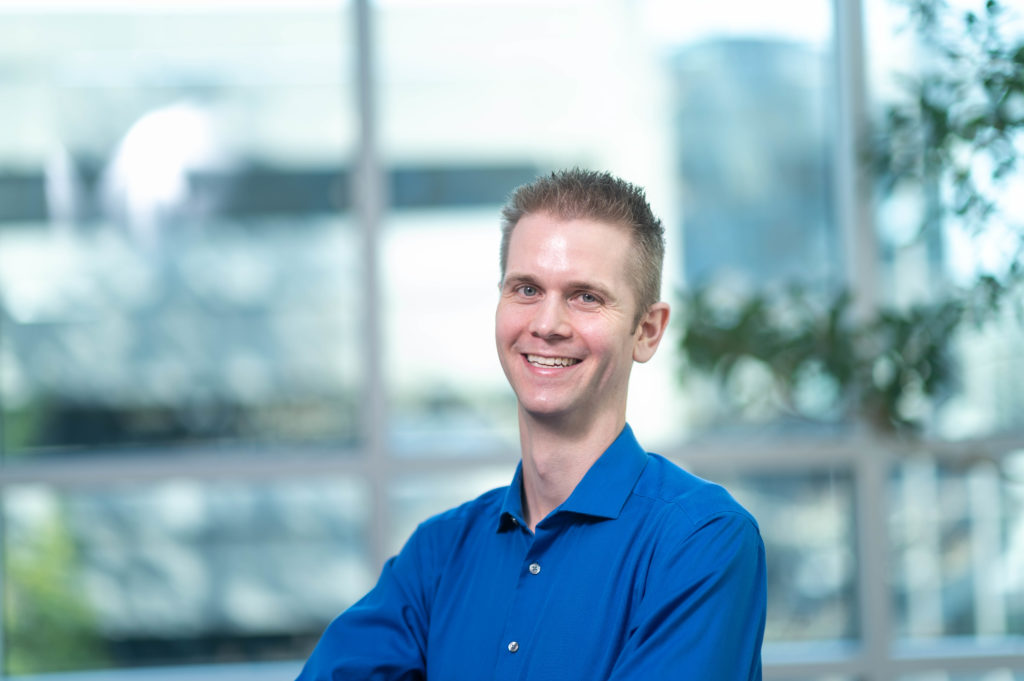
The de Boer Lab at SBME aims to solve the complex problem of determining when, where, and how genes will be expressed based solely on their DNA sequence. Dr. Carl de Boer and Abdul Muntakim Rafi recently led a global effort to advance computational models that predict gene expression based on short regulatory DNA sequences. The effort was featured in Nature Biotechnology.
In recent years, scientists (including those in the de Boer Lab) created technology for measuring the expression activity of DNA sequences in a controlled context, which produces ideal data using machine learning to learn sequence-expression logic. However, the general trend in the field has been to develop new models for new datasets, making it unclear whether a model’s improved performance is due to a better architecture or simply superior training data. The lack of proper benchmarking means that models aren’t directly comparable to each other.
To address this issue, the team set out to design a gold-standard dataset for sequence-expression models. They measured the expression of millions of randomly generated promoter DNA sequences in yeast and designed a set of sequences that would test the models’ performances in different way and tested their ability to predict differences in expression for sequences when a sequence is mutated. This is a critical challenge for the field as these kinds of mutations are important in many diseases.
Following the creation of this dataset, the team organized the Random Promoter DREAM Challenge, bringing together over 300 researchers from academia and industry around the world. The team collaborated with Google TPU Research Cloud to provide computational resources to all participants, ensuring an equitable competition for everyone involved. Participants competed for dominance on a leaderboard over the course of the summer in 2022 and, at the end, submitted their best models.
After the challenge, the team’s primary goal was to systematically evaluate how different neural network architectures and training strategies affect the performance of genomics models. They developed a framework called ‘Prix Fixe’. Just like a prix fixe menu, they were able to make models by selecting one of each module type from the top-performing submissions. By systematically testing all combinations of these components, the team identified which architectural and training choices were best and made even better models. The models outperformed existing benchmarks on Drosophila and human genomic datasets. This demonstrated the models’ robustness and generalizability across different organisms.
This community effort was able to create high-quality, gold-standard datasets that are driving progress in genomics and significantly advanced the understanding of how to design effective neural network models for gene regulation. The competition brought to light the potential of collaborative challenges to accelerate scientific discovery.